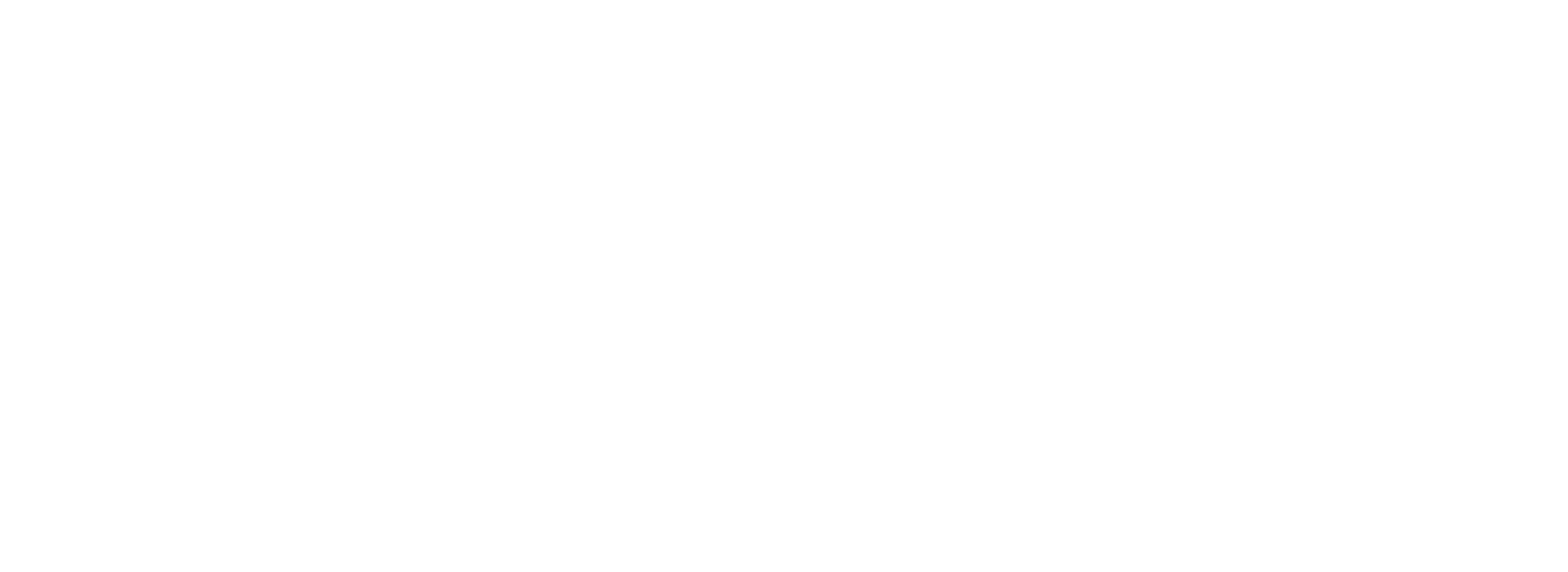
Sie benötigen eine leistungsstarke Software für komplexe Arbeiten im Bereich
Statistik, Grafik, Datenmanagement und automatisierte Berichterstellung?
Anders als viele andere Programme, bietet Ihnen Stata viele Vorteile. Überzeugen Sie sich selbst!
Stata Features
NEU in
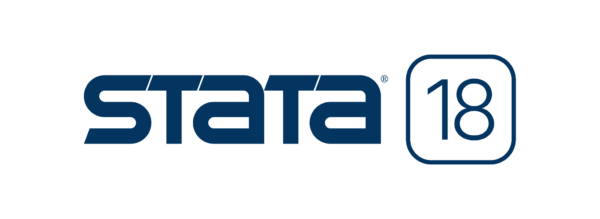
Bayesian model averaging (BMA)
Uncertain which predictors to use in your regression?
Use Bayesian model averaging to account for this uncertainty in your analysis. Explore influential models and predictors, obtain better predictions, and more.
https://www.stata.com/new-in-stata/bayesian-model-averaging-for-linear-regression/
Causal mediation analysis
Causal analysis quantifies causal effects. Causal mediation analysis disentangles them.
Are these effects mediated through another variable? Estimate direct and indirect effects. Calculate the proportion mediated.
https://www.stata.com/new-in-stata/causal-mediation-analysis/
Tables of descriptive statistics
When you publish your work, it is typical to include a table of descriptive statistics, commonly known as a „Table 1“; this provides your reader with some information about your sample. For example, you may want to present some demographics, such as average age and average income. You might also compare these characteristics across groups, such as regions or fields of occupation.
https://www.stata.com/new-in-stata/create-export-descriptive-statistic-tables/
Group sequential designs
Designing a clinical trial? In Stata 18, you can use the new gsbounds and gsdesign commands to calculate stopping boundaries for group sequential trials. What sample size is required for each interim analysis? Use gsdesign to find out.
Group sequential designs (GSDs) are a class of adaptive design for clinical trials. In a GSD, the sample size is not fixed in advance; instead, preplanned interim analyses are conducted to allow early stopping for efficacy or futility. This is done by defining stopping boundaries that control the familywise error rate. gsdesign calculates stopping boundaries and sample sizes for interim analyses with tests of means, proportions, survivor functions, and even user-defined methods.
https://www.stata.com/new-in-stata/group-sequential-designs/
Robust inference for linear models
Stata 18 offers more precise standard errors and confidence intervals (CIs) for three commonly used linear models in Stata: regress, areg, and xtreg, fe.
Small number of clusters? Uneven number of observations per cluster? Use HC2 with degrees-of-freedom adjustment, option vce(hc2 …, dfadjust), or wild cluster bootstrap to obtain valid inference.
Multiple nonnested clusters? Use multiway clustering, option vce(cluster group1 group2 … groupk), to account for potential correlation of observations within different clusters.
https://www.stata.com/new-in-stata/robust-inference-for-linear-models/
Wild cluster bootstrap
Do your data have a small number of clusters or an uneven number of observations per cluster? Do you want to make inferences about parameters in a linear model? With the new wildbootstrap command, you can now use wild cluster bootstrap (WCB) in these situations.
https://www.stata.com/new-in-stata/wild-cluster-bootstrap-inference/
Estimation of flexible demand systems
Eight flexible demands systems in just one convenient command—demandsys! Choose from the Cobb–Douglas demand system, almost ideal demand system (AIDS), generalized AIDS, and more. Estimate the demand for a basket of goods. Compute expenditure and price elasticities to evaluate sensitivity to expenditure and price changes.
https://www.stata.com/new-in-stata/flexible-demand-system-estimation/
TVCs with interval-censored Cox model
Stata 17 introduced the stintcox command to fit genuine semiparametric Cox models to interval-censored event-time data. Stata 18 adds support for time-varying covariates (TVCs), commonly used in practice to record characteristics that change during follow-up. So, whether you have a biomarker variable or a policy variable that changes with time, you can now include them to model events of interest known only to lie within some time interval. For instance, the event may be a recurrence of cancer or a change in employment status recorded during one of the follow-ups. Both are examples of interval-censored event-time data, in which the event time is not observed exactly.
Use stintcox to create TVCs automatically by interacting existing covariates with specified deterministic functions of time or specify your own TVCs in the new multiple-record-per-subject data format. Use TVCs to test the proportional-hazards assumption with the Wald test or likelihood-ratio test. And incorporate TVCs in your predictions and plots of survivor and other functions.
https://www.stata.com/new-in-stata/tvc-with-interval-censored-cox/
Goodness-of-fit plots for survival models
Stata 18 provides the new estat gofplot command to produce goodness-of-fit (GOF) plots for survival models. You can use it after four survival models: right-censored Cox (stcox), interval-censored Cox (stintcox), right-censored parametric (streg), and interval-censored parametric (stintreg). Check model fit after stratified models or separately for each by-group.
GOF plots provide visual checks for how well the model fits the data. In survival analysis, these checks are based on so-called Cox–Snell residuals and the assumption that, if a model is correct, these residuals should have a standard exponential distribution. Visually, this assumption is assessed by plotting the residuals against their estimated cumulative hazard—the closer the plotted values are to the 45° line, the better the fit (Cox and Snell 1968).
https://www.stata.com/new-in-stata/gof-plots-for-survival-models/
Lasso for Cox proportional hazards models
If you have time-to-event data, also known as survival-time or failure-time data, and many predictors, check out the lasso cox and elasticnet cox commands. (And when we say many predictors, we mean hundreds, thousands, or more!) New in Stata 18, these commands expand the existing lasso suite for prediction and model selection to include a high-dimensional semiparametric Cox proportional hazards model.
After lasso cox and elasticnet cox, you can use stcurve to plot the survivor, failure, hazard, or cumulative hazard function or use any of the other postestimation tools available after lasso and elasticnet.
https://www.stata.com/new-in-stata/lasso-cox-proportional-hazards-models/
Heterogeneous difference in differences (DID)
When average treatment effects vary over time and over cohort, you can now use the new hdidregress and xthdidregress commands to estimate heterogeneous average treatment effects on the treated (ATETs). Use hdidregress with repeated cross-sectional data and xthdidregress with panel data. Choose from one of four estimators, including regression adjustment and inverse-probability weighting. Plot ATETs time profiles for each cohort with estat atetplot. Aggregate the ATETs within cohort, time, and exposure to treatment with estat aggregation. Explore more postestimation features.
https://www.stata.com/new-in-stata/heterogeneous-difference-in-differences/
Multilevel meta-analysis
You want to analyze results from multiple studies, in which the reported effect sizes are nested within higher-level groupings such as regions or schools. Stata 18 adds two new commands, meta meregress and meta multilevel, to the meta suite to perform multilevel meta-analysis and meta-regression. Include random intercepts and coefficients at different levels of hierarchy, and assume different random-effects covariance structures, including exchangeable and unstructured. Perform sensitivity analysis by placing various constraints on variance components. Assess heterogeneity. Predict random effects and their comparative and diagnostic standard errors. And more.
Multilevel meta-analysis is a powerful statistical tool to synthesize effect sizes with a hierarchical structure, such as in a meta-analysis exploring the impact of a new teaching technique on math testing scores in different school districts. Here the effect sizes are nested within schools that are themselves nested within districts. Multilevel meta-analysis allows us not only to determine the overall effect of the technique but also to assess the variability among the effect sizes at different levels of the hierarchy. This is important because studies within the same district are likely to be similar and thus potentially dependent, and ignoring this dependence may lead to inaccurate results. By properly accounting for the dependence among the effect sizes, we can produce more accurate inference and gain a better understanding of the impact of the teaching technique.
https://www.stata.com/new-in-stata/multilevel-meta-analysis/
Meta-analysis for prevalence
You asked, we listened! The meta suite now supports meta-analysis (MA) of one proportion, or prevalence. Multiple types of effect sizes, confidence intervals, and back-transformations are supported. All standard meta-analysis features such as forest plots and subgroup analysis are supported.
https://www.stata.com/new-in-stata/meta-analysis-prevalence-proportions/
Local projections for impulse–response functions
With impulse–repsonse functions, you can find out how a shock to one variable affects other variables over time. With local projections, you can estimate impulse–response functions directly using multistep regressions. Use the new lpirf command to estimate local projections, and graph or tabulate them with the irf suite.
https://www.stata.com/new-in-stata/local-projections-impulse-response-functions/
Model selection for ARIMA and ARFIMA
Want to find the best ARIMA or ARFIMA model for your data? Compare potential models using AIC, BIC, and HQIC. Use the new arimasoc and arfimasoc commands to select the best number of autoregressive and moving-average terms.
Researchers using autoregressive moving-average (ARMA) models must decide on the proper number of lags to include for the autoregressive and moving-average parameters in their models. Information criteria, which balance model fit against model parsimony, often guide the choice of the maximum number of lags.
arimasoc and arfimasoc assist in model selection by fitting a collection of autoregressive integrated moving average (ARIMA) or autoregressive fractionally integrated moving average (ARFIMA) models and computing information criteria for each model. arimasoc and arfimasoc compute the Akaike information criterion (AIC), the Bayesian information criterion (BIC), and the Hannan–Quinn information criterion (HQIC). The selected model is the one with the lowest value of the information criterion.
https://www.stata.com/new-in-stata/arima-arfima-model-selection/
RERI
How do exposures interact to increase risk? Do you suspect that interaction is additive? Use reri to find out. Three measures of two-way interactions are provided: RERI, AP, and SI. Many models that estimate RR are supported, including logistic, binomial generalized linear, and survival.
https://www.stata.com/new-in-stata/relative-excess-risk-interaction/#overview
Spline function generation
Often, we do not want to make functional form assumptions about the data we analyze. We may want to fit a regression of an outcome on a set of regressors and be agnostic about the functional form of the regressors. Spline basis functions are flexible approximations to the functional form of the regressors. We may also want to visualize the relationship between an outcome and a regressor or between variables. We may use splines to visualize this relationship without claiming linearity or other functional forms.
https://www.stata.com/new-in-stata/spline-function-generation/
Corrected AIC and consistent AIC
By popular request, the existing estat ic and estimates stats commands now support two new model-selection criteria: corrected Akaike information criterion (AICc) and consistent AIC (CAIC). The new option all displays all available information criteria. The new option df() specifies the degrees of freedom to compute the information criteria.
https://www.stata.com/new-in-stata/aic-corrected-consistent/
Instrumental-variables fractional probit model
Fractional outcomes are common. You might be modeling participation rates in a 401(k) pension plan, the pass rate on standardized tests, expenditure shares, or the like.
Fractional response models are a flexible and intuitive way to model outcomes that lie between 0 and 1. They do not have the problem of linear models that will yield predictions outside 0 and 1 or the problem of log-odds models that are undefined at 0 and 1. Fractional response models can be fit using the fracreg command.
What if you are concerned that one or more of your model covariates are endogenous? With the new ivfprobit command, you can fit a model for a fractional dependent variable and account for endogeneity in one or more of the covariates.
https://www.stata.com/new-in-stata/fractional-probit-model-instrumental-variable/
Instrumental-variables quantile regression
When we want to study the effects of covariates on different quantiles of the outcome, we use quantile regression. But what if we suspect that a covariate is endogenous? The new ivqregress command models quantiles of the outcome and, at the same time, controls for problems that arise from endogeneity.
https://www.stata.com/new-in-stata/instrumental-variable-quantile-regression/
Stata graphs have an all-new style
You asked. We listened. Stata has an all-new default graph style.
Graph colors by variable
Want colors of the points in your scatterplot to reflect age groups? Or want the color of bars in your bar graph to reflect income level? Or want the colors of dots in your dot plot to reflect health status?
In Stata 18, the new colorvar() option allows many twoway plots to vary the color of markers, bars, and more based on the values of a variable.
https://www.stata.com/new-in-stata/graph-colors-by-variable/
Alias variables across frames
tata supports multiple datasets in memory; each dataset resides in a frame. In Stata 18, you can now work with variables from different frames as if they exist in one.
When datasets are related, you can link their frames by using the frlink command to identify the variables that match the observations in the current frame with observations in the related frame.
Alias variables, created by the new fralias add command, define references to variables in linked frames. These variables take up very little memory because the observations are actually stored in another frame.
Stata treats alias variables like any other variable in your dataset, with the exception that you are not allowed to change their values. For a given alias variable, if you change the corresponding variable’s values in the linked frame, the changed values are automatically available the next time you use the alias variable.
https://www.stata.com/new-in-stata/alias-variables-across-frames/
Saving, using, and describing a set of frames
You work with multiple datasets in memory, also known as frames. When those datasets are related—perhaps they are used in the same project or linked to each other—you can now bundle them in a frameset. Save all of the datasets in one file. Use them all together later.
Boost-based regular expressions
Regular expressions are powerful tools for working with string data. Stata’s regular expressions have become even more powerful, with more features, in Stata 18.
https://www.stata.com/new-in-stata/regular-expression-functions-boost/
Vectorized numerical integration
Numerical integration is used in many computations of integrals when the analytic solutions are not available or difficult to calculate. Vectorized numerical integration approximates a vector of univariate numerical integrations simultaneously.
Mata’s new class, QuadratureVec(), is functionally the same as Quadrature(), except that it handles a vector of integration problems more conveniently. More precisely, QuadratureVec() approximates a vector of univariate integrals numerically by the adaptive Gauss–Kronrod method (the adaptive Simpson method is also provided for comparison).
QuadratureVec() is used in the same way as Quadrature() in only four steps, namely, creating an instance of the class QuadratureVec(), specifying the evaluator functions, setting the limits, and performing the computations.
https://www.stata.com/new-in-stata/vectorized-numerical-integration/
New reporting features
Reproducible reports allow us to streamline the process of presenting our findings as our analyses change. Whether the direction of our work changes or we implement feedback from our peers, creating a report with the findings of our research is rarely a one-time task. Stata’s reproducible reporting features allow us to easily modify and adapt our reports as our analyses change.
In Stata 18, we have added features for putdocx and putexcel that allow you to further customize your reproducible reports. Now you can include headers, footers, and page breaks with putexcel. You can also freeze a row or column in the worksheet; this allows you to maintain information from that row or column in view, while scrolling through the rest of the sheet. Additionally, you can create a named cell range to simplify working with formulas. We have also added support for bookmarks with putdocx; simply format your text as a bookmark, and link to it as needed. Additionally, when adding an image to a .docx file, you can now specify alternative text for the image to be read by voice software.
The dtable command is another new reporting feature in Stata 18. Learn more here about how you can use it to easily create a table of descriptive statistics, often called a „Table 1“.
Do-file Editor enhancements
Automatic backups and syntax highlighting of user-defined keywords are now available in Stata’s Do-file Editor.
Data Editor enhancements
The Data Editor has been rewritten for Stata 18. Users of previous versions of Stata will find the new Data Editor very familiar, but Stata 18 includes a host of new features, including pinnable rows and columns, resizable cell editors, tooltips for truncated text, the ability to show variable labels in the column headers, and proportional-width fonts.
More new features in Stata 18
Einsatzbereiche
Forscher verschiedenster Disziplinen setzen seit mehr als 30 Jahren auf Stata Software. Und das mit Erfolg, denn Stata bietet ihnen alles, was sie im Bereich Datenmanagement, Visualisierung, Statistik und automatisierte Berichterstellung benötigen.
Womit beschäftigen Sie sich? Finden Sie heraus, was Stata für Sie tun kann:
Warum Stata?
Hochwertige Grafiken
Mit Stata erstellen Sie qualitativ hochwertige Diagramme für Publikationen und interne Dokumente. Sie können jederzeit zwischen vordefinierten oder selbst eingestellten Diagrammtypen wählen. Mit dem integrierten Grafik-Editor können Sie die Diagramme per Mausklick individualisieren und Titel, Notizen, Linien, Pfeile und Text hinzufügen. Mit selbstgeschriebenen Scripten lassen sich tausende Grafiken erzeugen (reproduzierbar) und exportieren – als EPS oder TIF für Publikationen, als PNG für das Internet oder auch PDF.
Schnell, genau und einfach zu benutzen
Dank seiner benutzerfreundlichen Oberfläche und der intuitiven Befehlssyntax ist Stata besonders einfach zu bedienen. Stata liefert genaue statistische Ergebnisse in Hochgeschwindigkeit. Die Ergebnisse können Sie mit minimalem Aufwand dokumentieren und für Veröffentlichungen reproduzieren. Stata’s Online-Hilfe und weitere Online-Ressourcen unterstützen bei der Auswertung komplexer Fragestellungen.
Umfassendes Datenmanagement
Die Datenmanagement-Befehle in Stata ermöglichen dem Anwender die vollständige Kontrolle aller möglichen Datentypen: Man kann Datensets kombinieren und umordnen, Variablen verwalten und statistische Auswertungen über Gruppen oder Replikate hinweg ausführen. Stata beinhaltet zudem erweiterte leistungsstarke Werkzeuge für die Arbeit mit speziellen Daten wie Survival-, Zeitreihen-, Panel-/Longitudinal-, Multiple-Imputation-, kategoriale und Survery-Daten.
Betriebssystem-übergreifend einsetzbar
Stata läuft unter Windows, Mac und Unix-Betriebssystemen (inkl. Linux). Stata-Lizenzen sind plattform-unabhängig. Stata-Datensätze, Programme und andere Daten können betriebssystem-übergreifend ohne weitere Übersetzung genutzt werden. Datensätze aus anderen Statistikprogrammen, Tabellen und Datenbanken lassen sich schnell und einfach importieren.
Matrix-Programmiersprache: Mata
Sie müssen zwar nicht programmieren, um Stata verwenden zu können, dennoch bietet Stata Ihnen mit Mata eine schnelle und vollständige Matrix-Programmiersprache. Mata ist beides – eine interaktive Umgebung zur Bearbeitung von Matrizen und eine komplette Entwicklungsumgebung zur Erstellung von kompiliertem und optimiertem Programmcode. Mata beinhaltet spezielle Features, um Paneldaten zu verarbeiten und Operationen auf reellen oder kompletten Matrizen durchzuführen. Mata unterstützt eine objektorientierte Programmierung und bietet eine vollständige Integration mit allen Stata relevanten Funktionalitäten und Charakteristika.
Erweiterbar
Stata ist so einfach zu programmieren, dass Entwickler und Nutzer täglich neue Features hinzufügen, um die Anforderungen der Anwender zu erfüllen. Neue Features und offizielle Updates können kostenlos aus dem Internet mit einem einzigen Klick heruntergeladen und installiert werden. Das quartärlich erscheinende Stata Journal informiert Sie über neue Features und aktuelle Anwendungsbeispiele. Über die Statalist, einem unabhängigen Listserver, tauschen Stata-User Informationen und Programme aus.
Ressourcen
Editionen

Stata BE
Basic Edition für mittelgroße Datensätze
Mit Stata BE lassen sich Datendateien mit bis zu 2.048 Variablen auswerten. Die Anzahl der Beobachtungen ist nur durch die RAM-Größe Ihres Rechners begrenzt. Stata BE kann maximal 798 unabhängige Variablen in einem Modell verarbeiten.

Stata SE
Standard Edition für größere Datensätze
Stata SE und Stata BE unterscheiden sich vor allem hinsichtlich der Größe der analysierbaren Datendateien. Mit Stata SE lassen sich Datendateien mit bis zu 32.767 Variablen und maximal 10.998 unabhängige Variablen in einem Modell verarbeiten.

Stata MP
Die schnellste Edition mit der die größten Datensätze analysiert werden können
(Für Quad-Core-, Dual-Core- und Multicore- / Multiprozessor-Computer)
Stata MP ist die schnellste und leistungsstärkste Stata-Version. Stata MP läuft auf Dual-Core-Computern (Intel Core 2 Duo, i3, i5, i7, i9, Xeon, Celeron, AMD X2) rund 40% schneller. Sogar 78% Beschleunigung erreicht Stata MP bei den zeitaufwändigen Estimation-Befehlen. Noch schnellere Analysen erzielt Stata MP auf Multi-Core- und Multiprozessor-Systemen. Stata MP verarbeitet Datendateien mit bis zu 65.532 Variablen und erstellt Modelle mit maximal 120.000 unabhängigen Variablen.
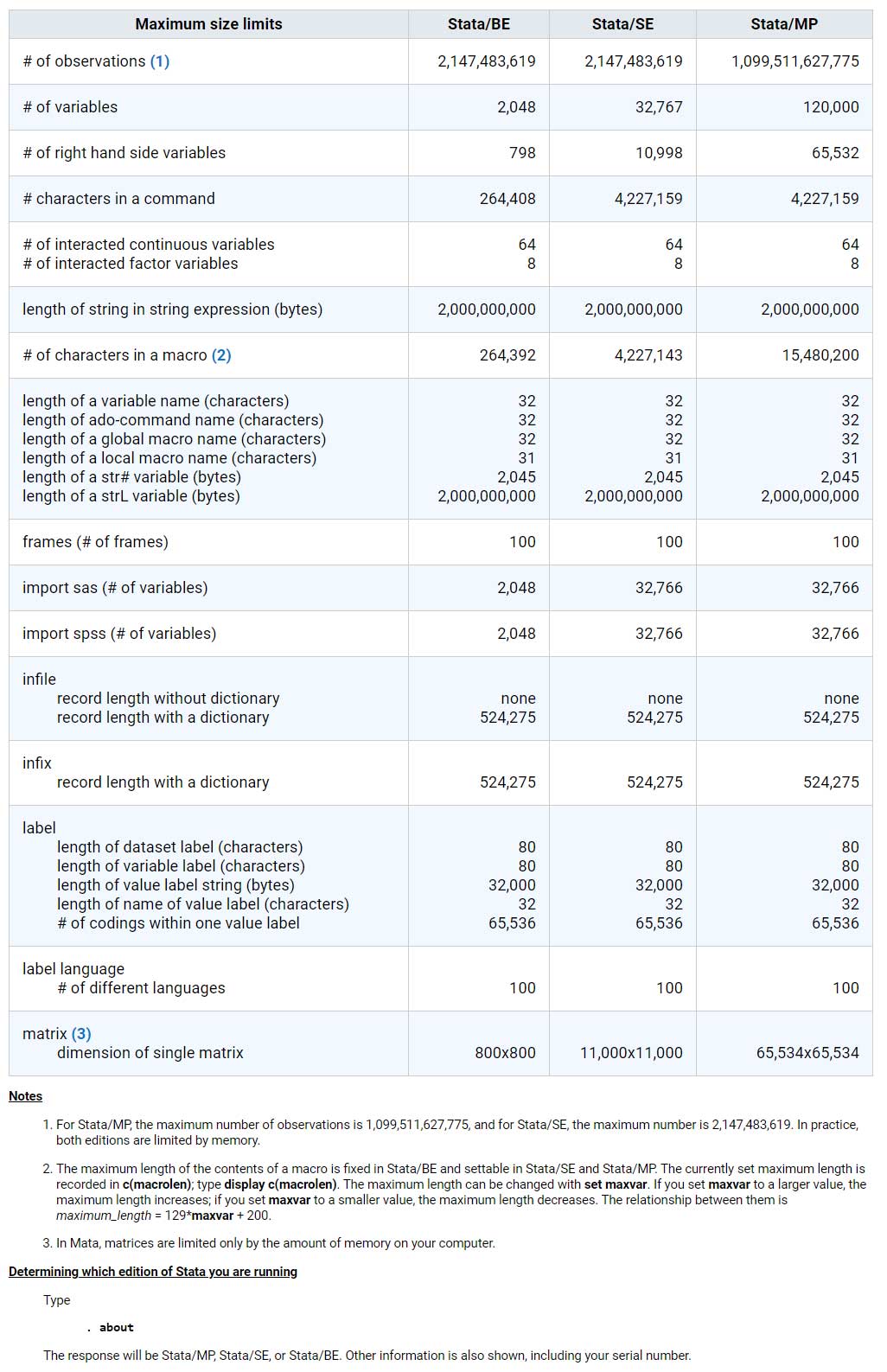
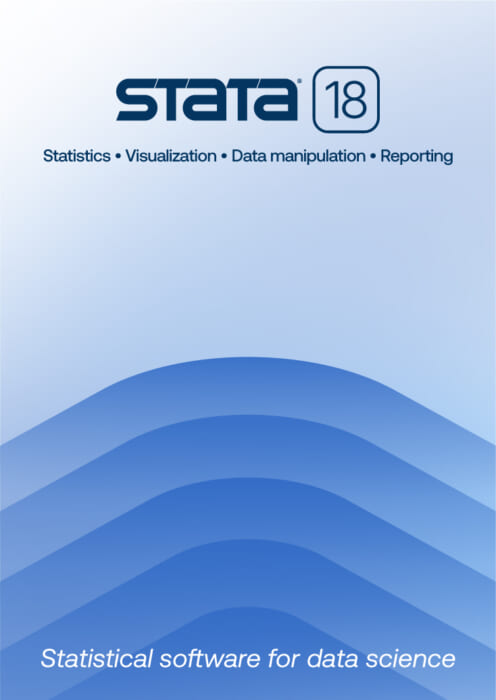
Systemanforderung
Stata for Windows
- Windows 11*
- Windows 10*
- Windows Server 2022, 2019, 2016, 2012R2*
* 64-bit for x86-64 made by Intel® and AMD
Stata for Mac
- Mac with Intel processor or Apple Silicon
- macOS 10.13 (High Sierra) or newer for Macs with Intel processors and macOS 11.0 (Big Sur) or newer for Macs with Apple Silicon
Stata for Linux
- 64-bit (x86-64)
- Minimum requirements include the GNU C library (glibc) 2.17 or better and libcurl4
Hardware-requirements
- Minimum of 1 GB of RAM für IC, 2 GB RAM für SE, 4 GB RAM für MP
- Minimum of 2 GB of disk space
- Stata for Unix requires a video card that can display thousands of colors or more (16-bit or 24-bit color)
Hardware-Empfehlungen für Dual-core-, Multi-core oder Multiprozessor-Computer
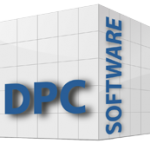
DPC Software GmbH
Offizieller Stata Distributor
für Deutschland, Niederlande, Österreich, Tschechien und Ungarn
Preise
Stata Testversion
Überzeugen Sie sich selbst und fordern Sie Ihre eine Stata Testversion von kostenlos für 30 Tage an.
Ihre Anfrage
Erhalten Sie ein unverbindlichen Angebot. Zugeschnitten auf Ihren Bedarf und Ihr Unternehmen.
Onlineshop
Kaufen Sie Stata für akademische oder nichtakademische Zwecke. Wir bieten auch spezielle Rabatte für Studenten über unseren Online-Shop an